This is excerpted from our Ebook, “Unleash the Power of Generative AI for Customer Journey Orchestration.”
Artificial Intelligence (AI) is enabling powerful advances across every industry and helping to solve many complex challenges and driving improved business results. In banking, for example, financial institutions are using AI to strengthen predictive analytics, automate repetitive tasks, improve voice recognition and combat fraudulent transactions. The healthcare industry is using AI to fastrack disease detection, provide personalized treatment plans and automate diagnostics, and retail organizations are using AI to improve and automate inventory management, among a host of other applications.
At the core of all these applications is the growing importance of AI in supporting the customer experience. With AI, businesses can provide personalized, efficient and frictionless interactions with customers at scale - a requirement for organizational success in a world where consumers are accustomed to real-time service and support across their channels of choice. However, the successful implementation of AI as a value driver requires careful thought and consideration of customer needs and expectations.
The Value of Generative AI for CX
In the lightning-fast business world of today, customer experience (CX) is a make-or-break factor for success. Businesses, regardless of size, need to understand their customers' journeys, scale them up, automate tasks, empower employees to respond in real-time, and connect partners to these journeys to drive customers towards completion.
AI is revolutionizing the way organizations approach CX management, providing them with the tools and insights they need to deliver personalized and connected experiences to customers.
Before we dive into the value of AI in CX, it's important to understand what AI is and how it works.
Artificial Intelligence: The 101
The first thing most people think about when they hear “AI” is sentient robots ready to take over the world. The reality, however, is far less eventful.
AI refers to computational technologies that can perform tasks that typically require human input by mimicking aspects of human intelligence, including learning, reasoning and self-correction. AI includes many subfields, each focused on solving specific problems. Companies leverage AI to automate tedious tasks at scale so that employees can focus their time on innovation and problem solving.
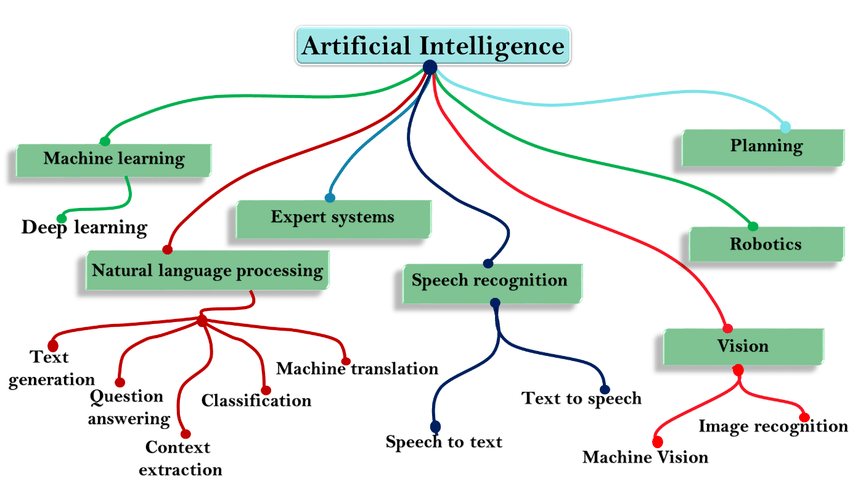
Main Subfields of AI
- Machine Learning (ML): Trains computers to learn from data to identify patterns and make predictions based on new data. ML technologies are used across a host of applications including image and speech recognition, and generative modeling.
- Analytics: Uses computational algorithms and statistical techniques to discover, interpret, analyze and communicate meaningful patterns and insights within large amounts of data. Analytics also pinpoints outliers, recognizes changes and identifies finding actionable business insights. Analytics is generally categorized into descriptive analytics, predictive analytics and prescriptive analytics.
- Natural Language Processing (NLP): Enables machines to understand, interpret, and generate human language. NLP technologies are used in several applications including sentiment analysis and chatbots.
- Expert Systems & Planning: Mimics the decision-making abilities of human experts. Expert systems are typically used in applications such as financial planning, legal decision-making and medical diagnosis. Planning focuses on the automated synthesis and optimization of action sequences to achieve a specific goal. Planning is used in a range of applications across industry to help agents make decisions about next best actions to take.
- Image Processing: Focuses on the analysis, manipulation and understanding of digital images to extract meaningful information from images/videos, improve image quality and enable automated interpretation of visual content.
- Speech Recognition: Involves recognizing and transcribing human speech into written text.
- Robotics: Involves designing, building, and programming robots to perform various tasks. Robotics technologies are used in a variety of applications across industries, including manufacturing, logistics and healthcare.
- Computer Vision: Enables machines to interpret and understand visual information from the world around them.
Three Main Categories of AI: Narrow, Broad and General
Narrow AI is focused on addressing very specific tasks based on “common knowledge” and limited to the tasks they are designed for. Examples of narrow AI include voice assistants like Alexa or Siri.
Broad AI refers to AI systems that can understand, learn and perform a wide range of tasks similar to what a human being can do. Examples include systems within a bank that can analyze the balance sheets of corporate customers to recommend optimal hedging strategies.
General AI goes beyond narrow AI and broad AI by achieving a level of intelligence that is comparable or surpasses human capabilities. It remains an aspirational goal for AI research and is far from reaching its potential.
For the enterprise, a fine balance between Narrow and Broad AI is optimal to support the vast majority of their use cases. Specifically, there are three core areas of AI within these domains that are critical to creating connected customer experiences:
- Cognitive Engagement: Designing and delivering new ways to improve customer engagement by turning digital experiences into more human-like natural conversations.
- Cognitive Insights and Knowledge: Augment and scale human knowledge and expertise, especially in environments where it is easy to become inundated with information.
- Cognitive Automation: Mimic human intelligence to facilitate complex business decisions quickly and efficiently.
According to PwC, AI is set to be the key source of transformation, disruption and competitive advantage in today’s fast changing economy, with the potential to contribute up to $15.7 trillion to the global economy in 2030. Similarly, Global Market Intelligence firm IDC predicts companies will use AI interactions and analytics to help automate customer engagement, eliminating over 40 percent of human touchpoints in marketing and sales.
What is Generative AI and Why is it Important for Businesses?
Generative AI is a subset of both Machine Learning and Natural Language Processing that focuses on generating new content or outputs based on patterns from a given dataset. Generative AI models are trained using large datasets to capture underlying structures in the data, often using deep learning techniques to capture complex patterns and generate high-quality data that resembles the training data. They are also trained to iteratively adjust to minimize the difference between generated and desired outputs.
With the release of ChatGPT in November 2022, the AI game changed entirely. Organizations are now looking at generative AI as a cost-efficiency engine across their business. Although there is a long way to go in this regard, especially when it comes to brand control, accountability and quality, the reality of today’s business landscape is simple: Organizations that fail to capitalize on the potential of generative AI risk falling far behind their competitors.

The CX Imperative
Today’s consumer has an abundance of options for almost every product imaginable, making it easy to switch brands with a few clicks of a button if their expectations are not met.
According to PwC’s Customer Loyalty Survey 2022, more than 25% of respondents had stopped using or buying from a business in the past year, largely because of poor experiences and subpar customer service. Similar numbers are echoed across other recent customer loyalty and satisfaction surveys, signaling an important reality: in a world where product differentiation is diminishing across competitors, CX is the ultimate differentiator when it comes to customer satisfaction, loyalty and business success.
Top Customer Experience Challenges
However, providing exceptional CX is not without its challenges. Some of today’s top CX challenges are:
- Data Silos: Managing and analyzing customer data is absolutely critical for delivering personalized experiences. However, data is holed up in different systems making it difficult to collect, process and use effectively.
- Poor cross-organization collaboration: Shared data visibility across third-party providers and partner organizations is either limited or non-existent, leading to collaboration breakdowns that ultimately impact the customer experience.
- Subpar Personalization: When team members can only see a sliver of the customer journey, companies are not able to track and take care of customers from end to end. That includes delivering personalized experiences in real time.
- Omnichannel Communication: Today’s customer interacts with businesses across multiple channels but employees and agents are not able to provide a seamless experience across all these channels. Customers are forced to repeat their question or concern multiple times, sometimes across multiple teams and departments - a frustrating experience for everyone involved.
- Varied Customer Issues: No two problems are the same. Customers present a wide range of issues and challenges, making it challenging for enterprises to anticipate and address them effectively.
- Proactive Issue Resolution: Thanks to the experiences provided by Amazon and Netflix, customers have become accustomed to their services understanding who they are, what they want and where they could run into an issue. For most businesses, anticipating and resolving customer issues before they become problems is far from reality.
- Employee Experience: Employees are rarely equipped with the tools and resources needed to deliver excellent customer experiences in the moment
- Incomplete Insights: Even though technology continues to develop at a rapid rate, businesses haven’t kept up by continually investing in tools that help them understand how to measure, analyze and improve their CX efforts.
Benefits of Generative AI for CX
When properly implemented, generative AI expands the scope of what can be automated across several fields. From this foundation, it can support businesses in overcoming the hurdles that prevent them from delivering outstanding CX:
- Data Management: Businesses can collect, process and use customer data more effectively. AI technologies can support the quick delivery of a unified customer view, enabling informed customer engagement across all channels.
- Shared Customer Visibility: Your customer experiences can depend on seamless collaboration with your partners. Save time by generating summaries and insights that span organizational boundaries without needing to review every note and transcript in detail.
- Personalization: Generative AI models offer the ability to quickly analyze customer data to provide recommendations, content, and offers tailored to individual preferences. It can also analyze past customer behaviors and purchase history to generate personalized recommendations and present them in a tone that resonates with the specific customer.
- Omnichannel Engagement: Generative AI can create interactive experiences through virtual assistants and interactive content to engage customers in providing real-time assistance and offering personalized support.
- Intelligent Issue Comprehension: AI systems can be trained on a diverse set of scenarios, enabling a better understanding of customer needs and providing appropriate resolutions.
- Proactive Issue Resolution: AI-powered tools can monitor customer interactions in real-time, identify patterns and trends, and analyze data that could indicate a problem long before it ever becomes one.
- Employee Experience: Generative AI can support employees by quickly pulling up and delivering relevant information, summarizing information, understanding sentiment and automating mundane, manual tasks that may add to employee burnout.
- Superior CX Analytics Tools: Identify patterns and trends in customer behavior quickly, allowing for more informed approaches towards CX improvements.
Read the next article: “Six Key Generative AI Use Cases for Journey Orchestration”
